Windfall AI System: Computer Aided Resource Detection System
Read More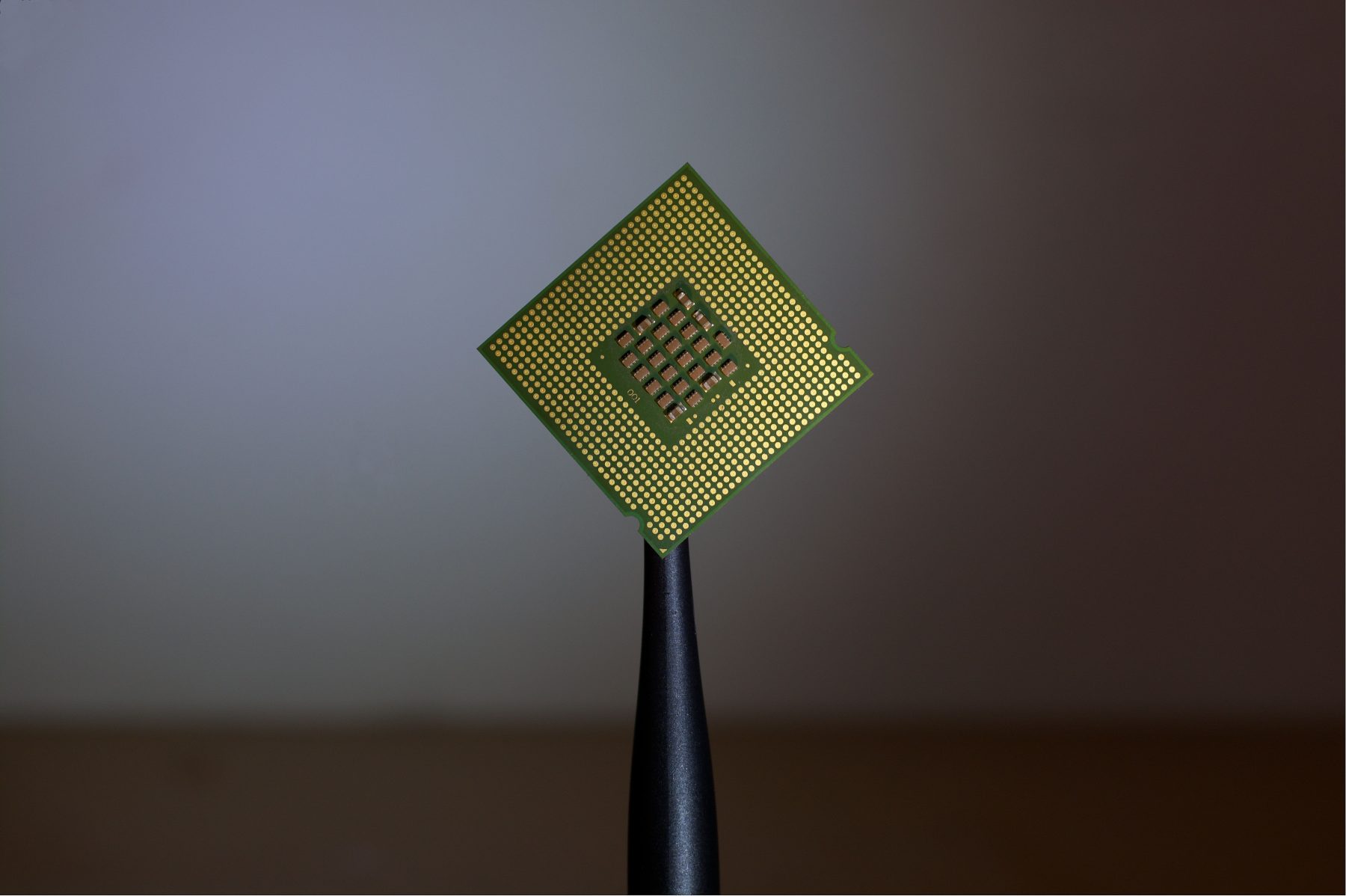
The Windfall AI System Platform
Pattern recognition algorithms identify predictive data sets.
Analysis of historic data can include:
- Geophysical surveys: MAG, EM, IP, Gravity, Radiometry, etc.
- Chemical Assay Results: Drill Holes & Surface Samples (Grab, Chip & Channel)
- Geochemical Surveys: Lake Bottom Sediment, Stream Sediment, Soil & Till
- Geology: Rock Type, Faults, Lineaments, Alterations, etc.
- Topography : Digital Elevation Models (DEM, DTM &SRTM)
- Satellite imagery: Lineaments/Stress, Alterations, Radiance, Geobotany, etc.
Breakdown into 2 databases allows for area-wide comparative analysis based on both known and unknown characteristics.
Unlike previous rule-based analytics, Windfall AI System is unbiased by any particular geologic model; learns and adapts and can make predictions on any mineral deposit type represented in the data set.
Result: An unprecedented and rapid ability to identify underground targets with high accuracy. Including all forms of mineral, groundwater and hydrocarbon deposits and even man-made buried explosive devices.
Windfall AI System locates mineral deposits in 4 easy steps
01Data Gathering & Process
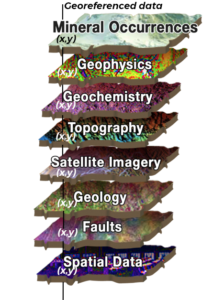
Windfall AI System manages this comprehensive & complex process, compiling and utilizing all available information modeling the target area of interest.
All the data is entered into Windfall AI System as geo-referenced data points. Each point in the database is linked to its own set of characteristics (variables) extracted from a variety of sources.
For example:
- Proximity to mineral occurrences / mineralized drill holes
- Geophysical surveys: MAG, EM, IP, gravity, radiometry
- Geochemical surveys: rock, soil, lake bottom, drill hole assays
- Satellite imagery
- Geological maps: rock type, alteration
- Digital elevation models
- Proximity to lithological contacts / specific intrusive suites
- Proximity to interpreted lineaments / mapped faults and shear zones
Windfall AI System identifies the positive points (drill holes and MDI’s) according to established thresholds for each of the mineralizations sought. By using a moving window, neighbouring patterns around each point are captured and expressed by new calculated variables for each primary exploration layer. In the analysis of each point in the database, the characteristics of all points within a specified distance (neighbourhood) are weighted into the evaluation of that point. Therefore the combination of their limited characteristics and their proximity to points with other significant characteristics similar to that of known positive points is identified.
02Model Set Up
The modeling analysis is based among other things, on the variable importance within the model comparisons. Variable importance is a difficult concept to define in general, because the importance of a given variable may be due to its (potentially complex) interaction with other variables.
In order to study the accuracy of predictions and to validate results, several methods are used and compared on each data block.
- Generated signature of known positive occurrences using multiple models that discriminate between the positive and unknown points using all existing information.
- Aggregate the different rules of all models by achieving a probability between 0 (unlike-positive) and 1 (like-positive) computed as the average of the different classification results. This probability represents the level of similarity of each point to the existing positive sites based on all variables employed in the modeling.
03Data Mining & Prediction
Windfall AI System classifies each new unknown point based on the rules of classification already generated: a point is considered as positive if its probability is higher than a specified threshold level.
The platform employs a validation learning algorithm using the same input data of the predictive algorithm to ensure that the statistical process is working properly and that the results intuitively make sense.
04Interpretation & Reporting
Investigation includes visually comparing target grids based on different models in order to identify target relevance and priorities.
Targets generated by Windfall AI System are evaluated in conjunction with all readily available geological data as part of the evaluation for the economic potential of a property, as well as the primary identification of exploration targets.